SNIa Hosts
Contact
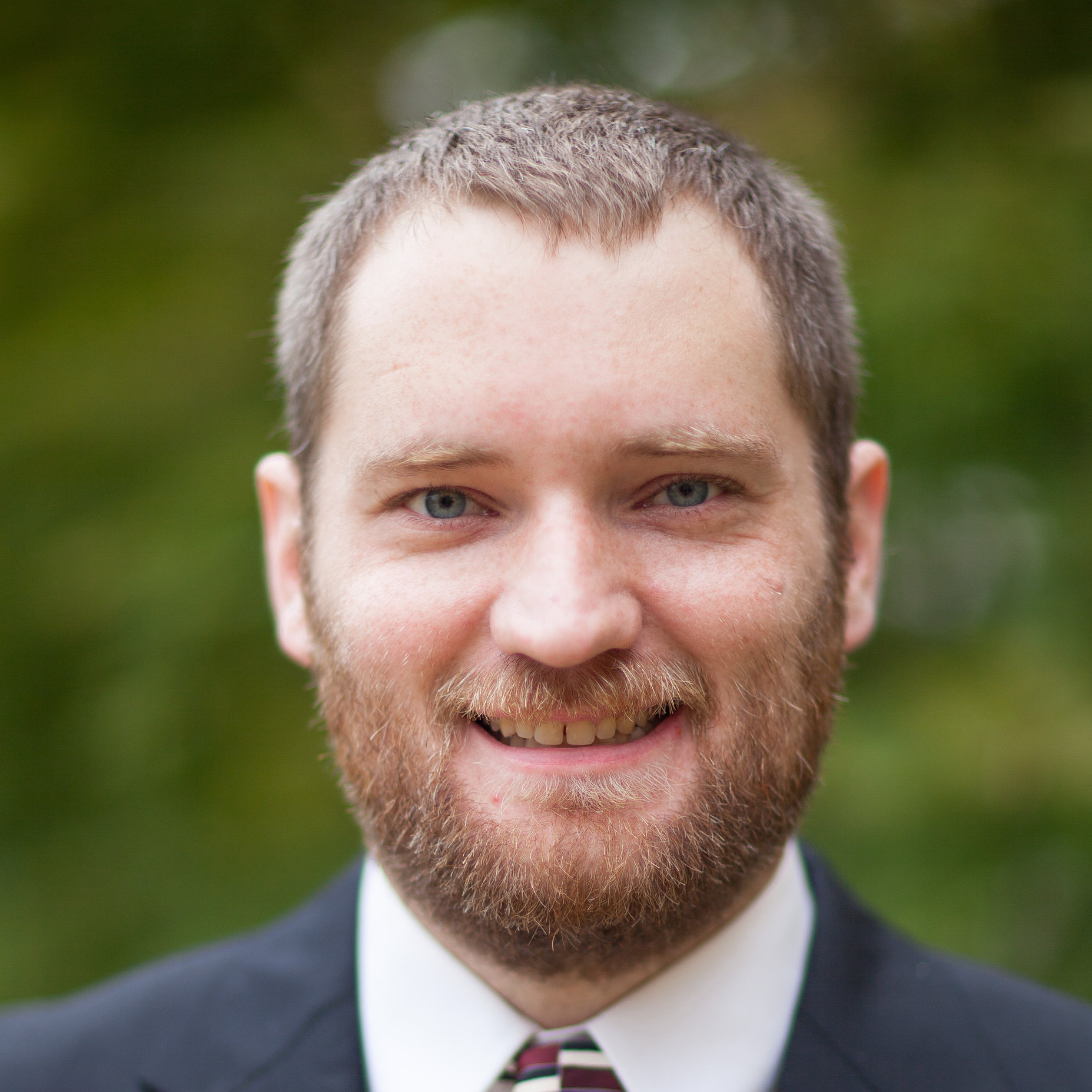
Benjamin Rose |
---|
University of Notre Dame |
Summary
Type Ia supernovae are distance indicators that have shaped the field of observational cosmology for decades. They are standardizable candles meaning their intrinsic brightness can be derived. These calibration corrections have historically been associated only with the light from the SNIa itself, now research is showing that the host galaxy may be affecting the peak luminosity of a Type Ia supernovae. This ancillary project will collect data from host galaxies in order to understand what may be affecting Type Ia supernovae.
Finding Targets
An object whose MANGA_TARGET3
or MNGTARG3
value includes one or more of the bitmasks in the following table was targeted for spectroscopy as part of this ancillary target program. See SDSS bitmasks to learn how to use these values to identify objects in this ancillary target program.
Program (bit name) | Bit number | Target Description |
---|---|---|
SN1A_HOST | 26 | SNIa host galaxies |
Description
Type Ia supernovae (SNIa) show a spread in the brightness of about 0.5 mag, but can be standardized by taking into account relationships like luminosity-decline rate and SNIa color to reduce the spread to 0.12 mag. Research over the past several years indicates that some of this remaining spread correlates with global host galaxy properties (e.g. Lampeitl, et al. 2010). Because this correlation can lead to systematic biases in cosmological measurements, there has has been an intense search for correlations between Hubble residuals (residuals from a best fit cosmology) and global host properties such as stellar mass, star-formation history, and metallicity (e.g. Gupta et al. 2011; Hayden, et al. 2013). The largest correlation with Hubble residuals occurs when looking at the total mass of the host, which is puzzling. Childress, et al. (2014) has proposed that the mass correlation is really an age effect.
In addition, the Hubble constant derived from Cepheids and SNIa appears to be in tension with the Hubble constant estimated from the cosmic microwave background experiments. The difference is now more than 3? in size making it either exciting or worrisome depending if the source is due to real physics or a bias in the measurements. A possible source of a bias is the SNIa/Cepheid scale which depends on empirical calibrations and already shows a systematic bias in the Hubble residuals with total host mass. In particular, the Cepheids used to calibrate the SNIa hosts are massive stars that are seen only in star-forming galaxies, while SNIa are seen in all stellar populations. Recently, Rigault, et al. (2013) found an intriguing distribution of Hubble residuals by looking at the surface brightness of H? emission at the locations of SNIa in the Supernova Factory sample. They measured the surface brightness of the H? in the host and at the site of the supernova and identified a set of outliers in the Hubble residuals. It appears these 0.2 mag outliers are found in regions of reduced star-formation. This is troublesome for calibrating SNIa with star-forming galaxies as these outliers may explode in the more quiescent parts of a galaxy. A Hubble constant measurement with a precision of 3% cannot tolerate contamination from a significant number of these apparent deviant SNIa.
This project will test the Rigault et al. result with a different dataset: a combination of the SDSS-II and Foundation supernova samples. MaNGA will allow measurement of the star-formation rate and metallicity at the supernova position and allow us to compare the local rate with the rest of the galaxy.
Target Selection
These galaxies were hosts to SNIa discovered by SDSS-II (Campbell et al. 2013) and the Foundation (Foley et al. 2018) data sets. These galaxies are close enough to measure local environment H? but are far enough away so that their motion is dominated by cosmological expansion. We have a goal of observing 60 targets that fit within the MaNGA sample criteria. At the end of the program, we will also search for any additional SNIa host galaxies observed by MaNGA but not requested by this program.
References
Campbell, et al. 2013, ApJ, 763, 88
Childress, et al. 2014, MNRAS, 445, 1898
Foley, et al. 2018, MNRAS, 475, 193
Gallagher, et al. 2008, ApJ, 685, 752
Gupta et al. 2011, ApJ, 740, 92
Hayden, et al. 2013, ApJ, 764, 191
Lampeitl, et al. 2010, MNRAS, 401, 231
Rigault, et al. 2013, A&A, 560, A66